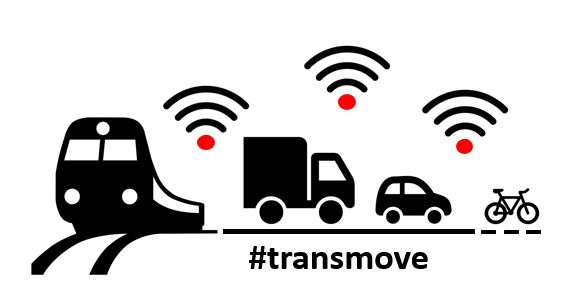
#transmove – AI-based traffic jam prediction
- contact:
- funding:
Landesbetrieb Straßen, Brücken und Gewässer (LSBG) Hamburg
- partner:
PTV Group
WPS - Workplace Solutions
TU München, Lehrstuhl für Verkehrstechnik
- start:
2022
- end:
2024
Problem Statement
In transport modeling, in recent years models have been developed that allow the mobility of entire regions to be represented at the individual level (agent-based models). However, due to their complexity, these models are often not used for strategic planning. Furthermore, the models usually only consider partial steps such as mode choice or assignment. As a result, the potential of these models is not fully exploited and planners cannot take advantage of the opportunities they offer, such as the effects of measures including individual effects.
Objective
Based on artificial intelligence, a forecasting tool is being developed to enable planners and other user groups to optimize traffic in a simple way. The project consists of a short-term forecast, which mainly relates to traffic flow and is intended to avoid congestion, and a strategic long-term forecast. A model is to be developed that integrates the two different planning levels.
Methods
The Institute for Transport Studies is in charge of the long-term forecast, in cooperation with the short-term forecast team (PTV and WPS). The basis for the long-term forecast is the agent-based travel demand model mobiTopp, which was created by the Institute for Transport Studies and TUM for the accompanying research for MOIA in Hamburg. This model is extended in the project in such a way that effects of traffic measures on a strategic planning level in the city of Hamburg are possible. The focus of the evaluations is the individual mobility behavior and the changes caused by the measures. The project combines different model frameworks (mobiTopp, MATSim, FleetPy and Visum). All project parts are based on the same database and a common platform for visualizing the results.